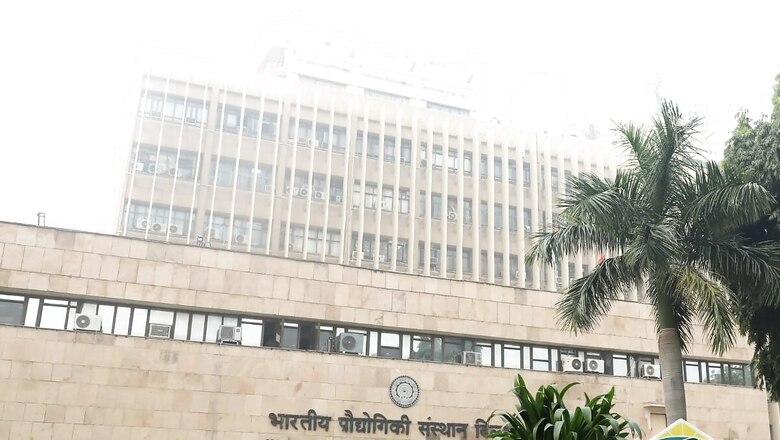
views
A machine learning model for monsoon rainfall prediction has been developed by researchers at the DST Centre of Excellence in Climate Modelling at IIT Delhi in association with IIIT Delhi, MIT in the United States, and JAMSTEC in Japan.
The All India Summer Monsoon Rainfall (AISMR) model developed by a research team led by Prof. Saroj K. Mishra of IIT Delhi predicts an AISMR of 790mm in the next monsoon season, indicating a regular monsoon for the nation in 2023.
The designed and tested AI/ML model has been shown to perform better than the country’s present physical models for monsoon forecasting. For the test period of 2002–2022, it has shown an outstanding forecast success rate of 61.9 per cent. The model’s ability to forecast the AISMR within +/-5% of the actual values measured each year is used to determine this.
In comparison to the highly resource-intensive procedure needed for conventional physical models, a small group of individuals running these models on a personal computer in just a brief amount of time can offer a more accurate monsoon rainfall forecast.
“This study holds immense significance for the entire country, as an accurate monsoon forecast well ahead of time is pivotal for making crucial decisions in various socioeconomic sectors, including agriculture, energy, water resources, disaster management, and health”, said Prof. Saroj K. Mishra
In order to make the data-driven methodologies more beneficial for regional applications, Prof. Mishra stated that they will be expanded to enable state-by-state monsoon rainfall forecasting.
The prediction is made using a model trained with historical AISMR data, Niño3.4 index data, and categorical Indian Ocean Dipole (IOD) data for the period 1901–2001.
Depending on the availability of the Nio3.4 index and IOD forecast, the prediction utilising the AI/ML model may be generated months in advance and updated appropriately based on how they are developing. As a result, data-driven models are less computationally costly, responsive to inputs, and better able to reflect the nonlinear relationships among the monsoon factors.
Comments
0 comment